The Importance of Machine Learning Data Labeling in Business Operations
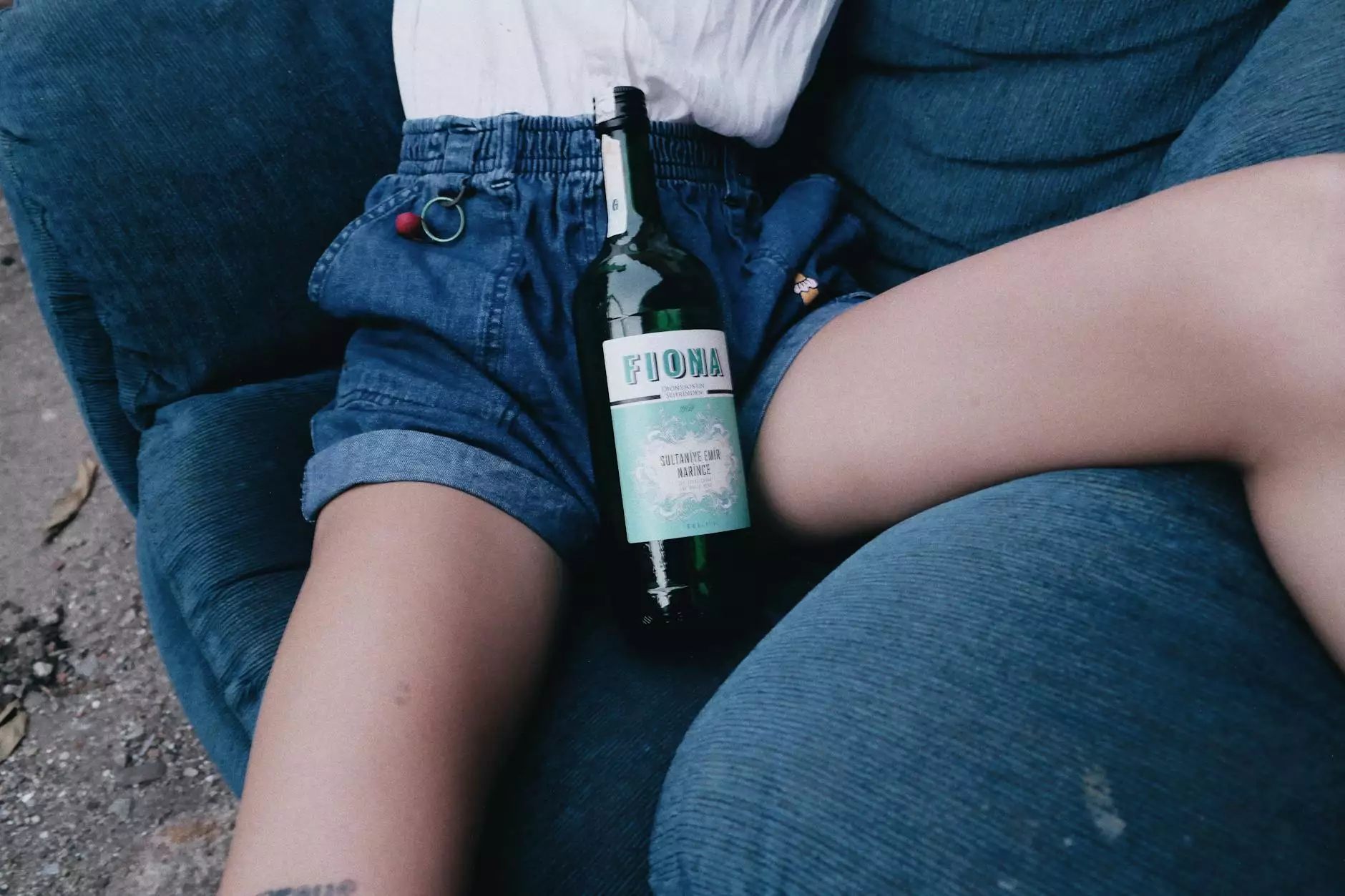
In today's digital age, business operations are heavily influenced by data. The ability to gather, analyze, and leverage data effectively shapes the competitive landscape of industries worldwide. One critical aspect of this data-driven environment is machine learning data labeling, which plays a pivotal role in enhancing the functionality of various business applications.
What is Machine Learning Data Labeling?
Machine learning data labeling is the process of annotating data so that machine learning models can learn from it. This involves assigning tags or labels to data points, allowing algorithms to recognize patterns and make predictions based on the input they receive. Companies, especially those in sectors like home services and keys & locksmiths, can greatly benefit from such technologies, as they allow for streamlined operations and enhanced service delivery.
The Relevance of Data Labeling in Home Services
The home services industry encompasses various fields, from plumbing and electrical services to locksmith solutions. With the advent of smart technologies, the demand for data-driven solutions has never been higher. Here’s how data labeling transforms the home services sector:
- Improved Customer Experience: By leveraging labeled data, businesses can personalize services, predicting customers' needs before they arise. For instance, a locksmith service can utilize machine learning to analyze patterns in locksmith requests, tailoring their marketing strategies accordingly.
- Efficiency in Scheduling: Using historical data, machine learning models can assist in optimizing service schedules, reducing waiting times, and enhancing customer satisfaction.
- Enhanced Safety and Security: In locksmith services specifically, the integration of machine learning algorithms can help identify potential risks and improve the security measures offered to clients.
Machine Learning Data Labeling in the Locksmith Industry
Locksmiths deal with sensitive information and critical services that directly impact customer safety. Here are specific areas where machine learning data labeling can be explored:
- Fraud Detection: By labeling historical data related to service requests, locksmith businesses can train machine learning models to recognize signs of fraud or scams.
- Predictive Maintenance: Annotated data can help identify which tools and equipment are likely to fail, allowing locksmiths to perform maintenance before critical failures occur.
- Intelligent Customer Support: Implementing chatbots that use labeled data can provide prompt responses to common customer inquiries, improving overall customer service efficiency.
The Steps to Effective Data Labeling
Successful machine learning data labeling requires a strategic approach. Here are the key steps to ensure effective labeling:
- Define Objectives: Clearly outline what you want to achieve with your data labeling efforts, whether it’s for predictive models or customer insights.
- Choose the Right Tools: Selecting appropriate labeling tools or platforms can make the process more efficient. Consider platforms that integrate seamlessly with your existing data workflows.
- Engage Skilled Annotators: Having trained personnel for labeling tasks ensures accuracy and reliability in the labeling process, which is vital for effective model training.
- Quality Control: Implementing checks to verify the accuracy of labeled data is crucial. Regular audits can help maintain a high standard of data integrity.
- Continuous Improvement: Machine learning is an evolving field. Keeping your models updated and retraining them with new data helps adapt to changing trends and customer preferences.
Benefits of Machine Learning Data Labeling for Businesses
The advantages of implementing machine learning data labeling in business processes extend beyond mere operational efficiency. Below are some prominent benefits:
- Data-Driven Decision Making: With labeled data, business leaders can make informed decisions backed by analytics rather than intuition.
- Automation: Many manual processes can be automated through machine learning algorithms, saving time and resources while improving accuracy.
- Competitive Advantage: Access to insightful data can give businesses a leg up on competitors who are not utilizing these technologies.
- Scalability: Implementing machine learning solutions allows businesses to scale operations quickly without a corresponding increase in manual labor.
Real-World Examples of Data Labeling Impact
To understand the tangible benefits of machine learning data labeling, let’s delve into some real-world examples:
Case Study: Home Services Company
A home services company invested in data labeling to improve service recommendations. By analyzing customer interactions and service histories, the company trained its machine learning models to suggest services tailored to individual needs. This led to a 20% increase in upsells and significantly improved customer satisfaction ratings.
Case Study: Locksmith Business Transformation
A locksmith business adopted machine learning to streamline their emergency response times. By labeling data from emergency calls and service outcomes, they were able to predict peak times and allocate resources efficiently, resulting in a 15% reduction in response times and heightened customer trust.
Challenges in Machine Learning Data Labeling
Despite its many benefits, machine learning data labeling is not without challenges. Some of the common obstacles businesses may face include:
- Cost: High-quality data labeling can require significant investment in both tools and skilled labor.
- Data Privacy Concerns: Handling sensitive information demands rigorous compliance with data protection laws, which can complicate data labeling efforts.
- Scalability of Labeling Efforts: As the volume of data increases, maintaining consistent labeling quality can become increasingly challenging.
The Future of Machine Learning Data Labeling in Business
As technology continues to advance, the role of machine learning data labeling in business ecosystems is expected to grow. Anticipated trends include:
- Automated Data Labeling: The development of AI-powered tools that can assist in or automate parts of the data labeling process, increasing efficiency and reducing costs.
- Augmented Decision Making: Machine learning models will continue to become more sophisticated, enabling better decision-making processes within various industries.
- Integration with IoT: As Internet of Things (IoT) devices proliferate, annotated data will be critical for analyzing vast amounts of information generated by these devices.
Conclusion: Harnessing the Power of Machine Learning Data Labeling
In conclusion, machine learning data labeling stands as a cornerstone in the functionality and efficiency of modern businesses, particularly in the sectors of home services and locksmithing. By understanding how to implement effective data labeling strategies, companies can unlock significant improvements in customer satisfaction, operational efficiency, and ultimately, profitability.
The future is bright for businesses that are willing to embrace the power of data. In an era where agility and precision are paramount, investing in machine learning data labeling not only keeps businesses ahead of the competition but also equips them to articulate customer needs and provide tailored solutions with remarkable accuracy.