Enhancing Machine Learning with the Best Image Labeling Tool for Classification
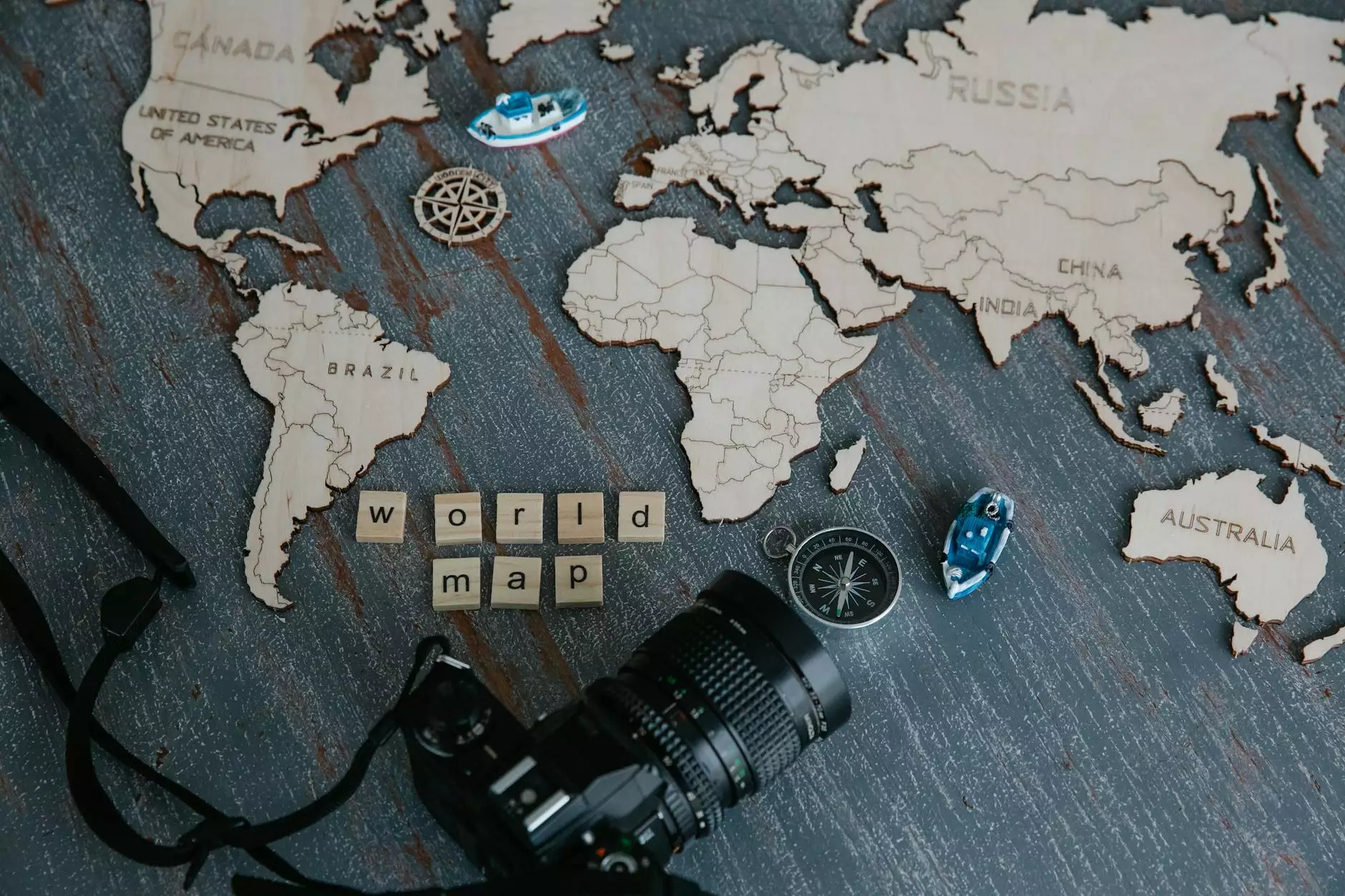
In the rapidly evolving domain of artificial intelligence and machine learning, data quality is paramount. One of the essential components contributing to high-quality data is image labeling, particularly for classification tasks. This article delves into the world of image labeling tools, focusing on their significance, best practices, and how a powerful image labeling tool for classification can elevate your projects. We'll also explore offerings from KeyLabs.ai, a leader in data annotation solutions.
Understanding the Basics of Image Labeling
Image labeling refers to the process of teaching a machine learning model to recognize and classify objects in images. This process involves annotating images with informative tags or labels, enabling algorithms to learn from these labeled datasets. The accuracy of a machine learning model is highly contingent upon the quality of the annotated data it is trained on. Thus, using a sophisticated image labeling tool for classification is crucial.
The Importance of Image Labeling in Machine Learning
Image labeling serves several purposes in the context of machine learning:
- Data Preparation: Labels create a structured dataset from raw images, making it usable for model training.
- Model Accuracy: High-quality annotations contribute directly to improved accuracy in predictions.
- Feature Recognition: Well-labeled images help algorithms recognize important features, essential for tasks ranging from object detection to facial recognition.
- Automation of Repetitive Tasks: Using image labeling tools can automate the tedious process of data annotation, saving time and resources.
- Label Variety: The necessity for diverse labels allows models to better generalize and perform in real-world scenarios.
Choosing the Right Image Labeling Tool for Classification
Choosing an effective image labeling tool for classification is crucial for the success of any machine learning project. Here are some factors to consider:
User Interface and Experience
A user-friendly interface can significantly enhance the efficiency of the labeling process. Tools that provide intuitive navigation and extensive functionality will likely reduce the learning curve for new users.
Customizability and Flexibility
Look for tools that offer customizable labeling options. The ability to adapt the tool to specific project needs ensures that all nuances of your classification task are accounted for.
Integration Capabilities
Integration with other data processing and machine learning frameworks is essential. A versatile image labeling tool for classification should seamlessly connect with data pipelines, ML models, and other essential platforms.
Quality Control Features
Incorporating quality checks and collaborative workflows can greatly improve annotation accuracy. Features such as consensus labeling, where multiple annotators review the same images, can help maintain high standards.
Scalability
The ability to scale up your labeling efforts as your dataset grows is crucial. Ensure the tool you choose can handle increasing volumes of images without compromising on performance.
Exploring KeyLabs.ai Image Labeling Solutions
KeyLabs.ai stands out in the market with its comprehensive range of data annotation tools. Here are some of the distinctive features that make it an exceptional choice for businesses looking for an advanced image labeling tool for classification:
Advanced Machine Learning Algorithms
KeyLabs.ai employs cutting-edge machine learning algorithms to assist users in labeling images effectively and efficiently. The algorithms improve over time, learning from user inputs and past annotations.
Collaboration and Team Management Features
Team collaboration tools allow multiple users to contribute to labeling projects, facilitating real-time feedback and consensus reviews. This collaborative approach promotes higher quality and faster turnaround times.
Highly Customizable Workflows
With KeyLabs.ai, users can design custom workflows tailored to their specific needs. This flexibility means you can focus on what matters most to your project without unnecessary distractions.
Robust Quality Assurance Protocols
KeyLabs.ai integrates extensive quality assurance measures, including automated checks and peer reviews, ensuring the accuracy and reliability of annotated data.
Seamless Integration with Popular ML Frameworks
The platform ensures easy integration with various machine learning and data processing frameworks, enabling smooth transitions from data collection to model deployment.
Best Practices for Image Labeling
To maximize the effectiveness of an image labeling tool for classification, consider the following best practices:
Define Clear Annotation Guidelines
Before starting the labeling process, establish clear guidelines and definitions for each label. Consistency in labeling is essential for training accurate models.
Invest in Training for Annotators
Training your annotators on the nuances of your labeling task can significantly enhance the quality of the annotations. Well-trained annotators are more likely to produce consistent and accurate labels.
Utilize Pre-labeling Features
Many modern image labeling tools offer pre-labeling features that use AI to suggest labels based on existing data. Utilizing this can save time and improve efficiency.
Conduct Frequent Quality Checks
Implement a routine for regular quality checks. Reviewing a sample of labeled images can help catch errors early and ensure the team adheres to the established guidelines.
The Future of Image Labeling Tools
The field of image labeling is continuously evolving, especially with advancements in AI and machine learning technologies. As we progress, some of the anticipated developments may include:
- Increased Automation: Automation will further streamline the labeling process, reducing the need for manual input and speeding up project timelines.
- Enhanced Accuracy: With improvements in AI, tools will likely become even better at suggesting labels without human intervention, enhancing overall accuracy.
- Integration with Virtual Reality (VR) and Augmented Reality (AR): The merging of these technologies could lead to innovative applications for image labeling.
- Data Privacy and Security Enhancements: As privacy concerns grow, tools will become increasingly adept at secure data handling and compliance with regulations.
Conclusion
In conclusion, the significance of using a highly efficient image labeling tool for classification cannot be overstated. As machine learning continues to influence various industries, the demand for accurate, high-quality labeled datasets will soar. Solutions provided by platforms like KeyLabs.ai offer the power, flexibility, and scalability needed to succeed in today’s competitive landscape. By implementing the best practices discussed and leveraging advanced tools, businesses can ensure their machine learning models are precise, reliable, and ready to meet the challenges of tomorrow.
Investing in an exceptional image labeling tool is not just a step toward better data annotation—it's a leap toward enhanced machine learning capabilities, driving innovation and fostering future growth.